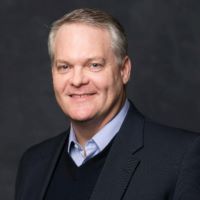
Professor
We combine the latest advances in satellite vegetation remote sensing and physical measurement with other publicly available data, processed using accessible machine learning techniques, to explore the potential to provide accurate, timely, and lower cost monitoring of key Feed the Future (FtF) outcome indicators such as asset poverty and nutritional status at the sub-national community level in low-income FtF countries. The resulting indicators enable higher frequency monitoring and adaptive targeting, as well as careful impact evaluation of FtF and other interventions when combined with rigorous research design around project or program participation
We thank the United States Agency for International Development for financial support under cooperative agreement # 7200AA18CA00014, “Innovations in Feed the Future Monitoring and Evaluation - Harnessing Big Data and Machine Learning to Feed the Future”. All data and written products are solely the authors’ responsibility and do not necessarily reflect the views of USAID or the United States Government.
Jiaming Wen, Philipp Köhler, Grégory Duveiller, Nicholas C. Parazoo, Troy S. Magney, Giles J. Hooker, L.Yu, Christine Y. Chang, Ying Sun
Remote Sensing of Environment
20 January 2020
L. Yu, Jiaming Wen, Christine Y. Chang, Christian Frankenberg, Ying Sun
Geophysical Research Letters
11 December 2018
Leiqiu Hu, Ying Sun, Gavin Collins, Peng Fu
ISPRS Journal of Photogrammetry and Remote Sensing
19 August 2020
Oz Kira, Ying Sun
ISPRS Journal of Photogrammetry and Remote Sensing
21 January 2020
Linden McBride, Christopher B. Barrett, Christopher Browne, Leiqiu Hu, Yanyan Liu, David S. Matteson, Ying Sun, and Jiaming Wen
Applied Economic Perspectives and Policy
July 2021
Binh Tang, Yanyan Liu, David S. Matteson
Applied Economic Perspectives and Policy
December 2021, forthcoming
Chris Browne, David S. Matteson, Linden McBride, Leiqiu Hu, Yanyan Liu, Ying Sun, Jiaming Wen, Christopher B. Barrett
PLOS ONE
June 2021, forthcoming
We focus on data from eleven USAID Feed the Future (FTF) priority countries: Bangladesh, Ethiopia, Ghana, Guatemala, Honduras, Kenya, Mali, Nepal, Nigeria, Senegal, and Uganda.
This dataset was created by fusing SIF retrievals from SCanning Imaging Absorption spectroMeter for Atmospheric CHartographY (SCIAMACHY) and Global Ozone Monitoring Experiment 2 (GOME-2) onboard MetOp-A developed at German Research Center for Geosciences (GFZ)
This dataset was created to provide researchers with a free and open source dataset for the estimation and prediction of poverty and malnutrition prevalence in developing nations.
Each datum in this dataset contains 5 outcomes (stunted, wasted, healthy, poorest, underweight_bmi) which measure poverty/malnutrition prevalence rates estimated via DHS surveys at a particular point in space and time. Estimation is done at the enumeration area level (roughly a village). Alongside these outcomes are many features which may be explanatory of these outcomes, sampled as close as possible to the enumeration area in space and time as possible, unless otherwise indicated (see paper). Data was extensively preprocessed, and used in our associated paper.
Chris Browne, David S. Matteson, Linden McBride, Leiqiu Hu, Yanyan Liu,Ying Sun, Jiaming Wen, Christopher B. Barrett "Multivariate random forest prediction of poverty and malnutrition prevalence," PLOS ONE, Forthcoming.
A guide to the Python code written by Chris Browne from Chris Browne, David S. Matteson, Linden McBride, Leiqiu Hu, Yanyan Liu,Ying Sun, Jiaming Wen, Christopher B. Barrett "Multivariate random forest prediction of poverty and malnutrition prevalence"," PLOS ONE, Forthcoming. and annotated code written by Medha Bulumulla.